Expert Insights: The Future of Machine Learning in Promoting Sustainable Energy
The Role of Machine Learning in Sustainable Energy
Machine learning (ML) is emerging as a pivotal technology in the quest for sustainable energy solutions. By leveraging vast amounts of data, ML algorithms can enhance energy efficiency, optimize resource utilization, and reduce carbon footprints. As the world shifts towards greener alternatives, understanding the potential of machine learning in this sector is crucial for future innovations.

Optimizing Energy Consumption
One of the most promising applications of machine learning in sustainable energy is optimizing energy consumption. By analyzing patterns in energy usage data, ML models can predict demand and adjust supply accordingly. This ensures that energy is used more efficiently, reducing waste and minimizing costs. Such technology is already being implemented in smart grids, where it helps balance load and prevent blackouts.
Moreover, machine learning can help identify inefficiencies in buildings and industrial processes, suggesting modifications that can lead to significant energy savings. These insights are invaluable for businesses aiming to reduce their environmental impact while maintaining operational efficiency.
Enhancing Renewable Energy Integration
The integration of renewable energy sources like solar and wind into existing power grids is a complex challenge due to their intermittent nature. Machine learning can play a crucial role in forecasting energy production from these sources, enabling better grid management and storage solutions. By accurately predicting weather patterns and energy output, ML helps in efficiently integrating renewables into the energy mix.
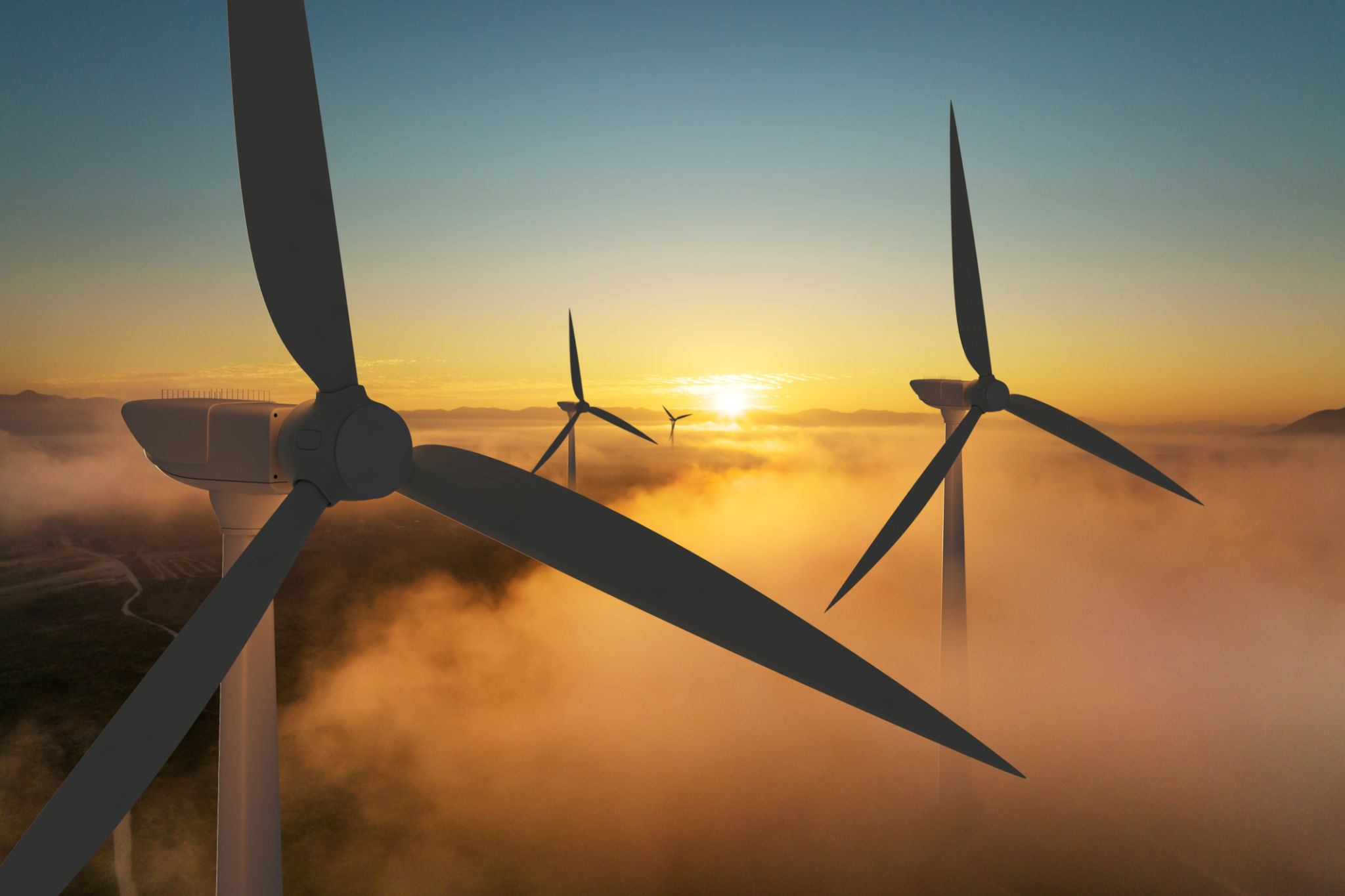
This capability not only helps stabilize the grid but also reduces reliance on fossil fuels, paving the way for a cleaner and more sustainable energy future. As renewable energy continues to grow, machine learning will be essential in managing this transition smoothly.
Improving Energy Storage Solutions
Energy storage is another critical area where machine learning offers significant benefits. Efficient storage solutions are necessary to ensure a steady supply of energy, especially when renewable sources are not producing at their peak. Machine learning algorithms can optimize battery management systems by predicting charge and discharge cycles, extending battery life, and improving performance.
By leveraging data from various sensors and monitoring systems, ML can enhance the reliability and efficiency of energy storage solutions, making them more viable for large-scale deployment. This advancement is vital for supporting the widespread adoption of renewable energy technologies.
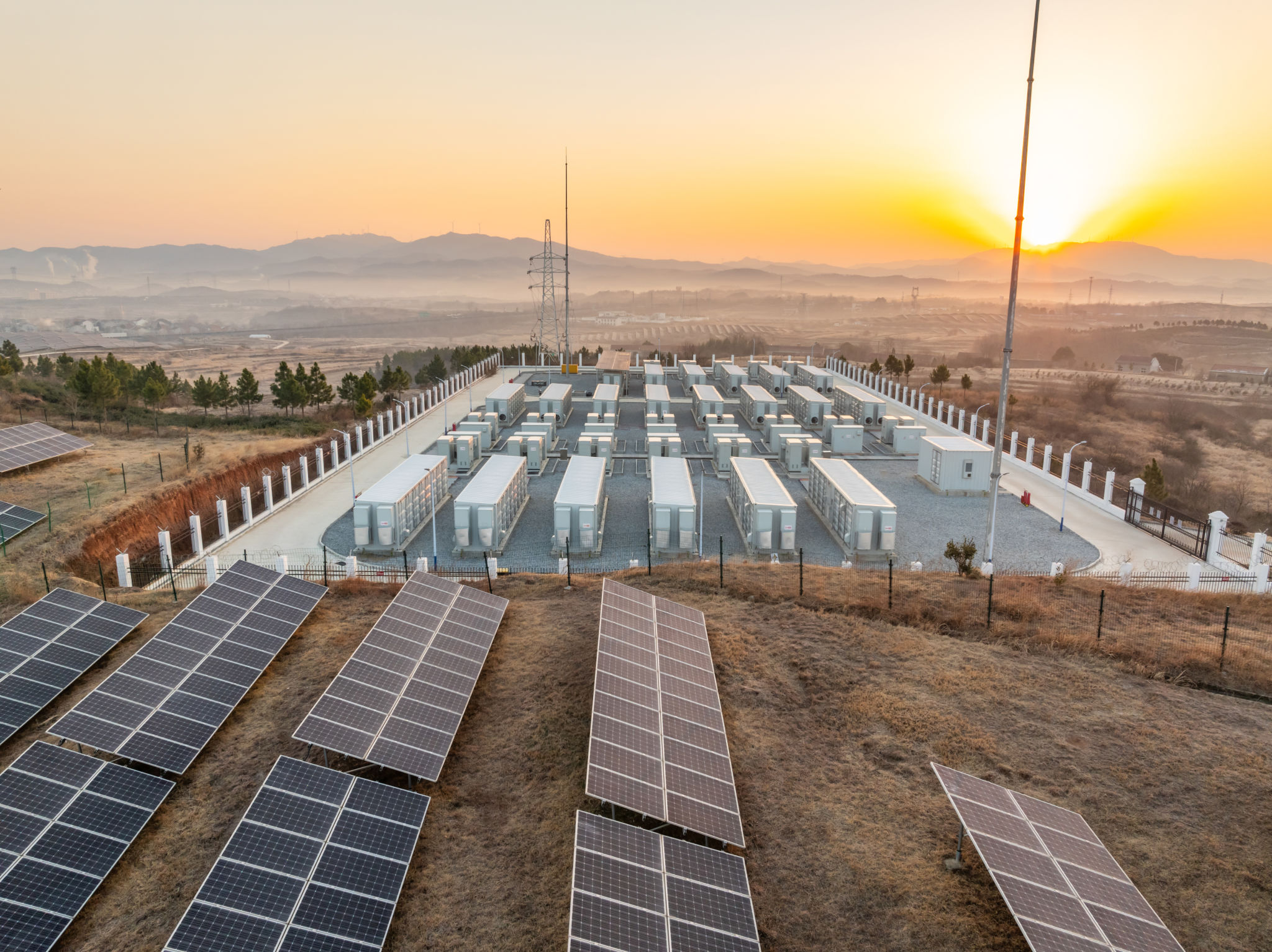
The Future of Machine Learning in Energy Systems
As machine learning technologies continue to evolve, their influence on sustainable energy systems will only grow stronger. Future developments could see even more sophisticated algorithms capable of real-time decision-making and autonomous system management. This could lead to fully automated grids that self-optimize for maximum efficiency and minimum environmental impact.
Ultimately, the future of machine learning in promoting sustainable energy is bright. By continuing to harness the power of data, we can unlock new possibilities and innovations that drive the world towards a more sustainable future. The key will be ongoing collaboration between technologists, policymakers, and industry leaders to ensure these advancements are implemented effectively and responsibly.